Colleagues, here is the
“ChatGPT for Language Translation and Summarization” of this new audio and ebook Week #4 on Amazon in the “Transformative Innovation” series for your reading-listening pleasure:
V - Using ChatGPT for Language Translation and Summarization
ChatGPT is a large language model that can be fine-tuned for a range of natural language processing tasks, including the translation of language and the summary of information. In this section, we will explain more in-depth how to utilize ChatGPT for language translation and summarization.
Language Translation
ChatGPT is a powerful language model that can be fine-tuned for various natural language processing tasks, including language translation. In this section, we will explain how to use ChatGPT for language translation in more detail.
The first step in using ChatGPT for language translation is fine-tuning the pre-trained model on a parallel corpus of text in two languages. A parallel corpus is a collection of texts in two languages: word-for-word, sentence-for-sentence, or paragraph-for-paragraph. These texts train the model to translate from one language to another. The fine-tuning process can be done using a technique called transfer learning, where the model is pre-trained on a large corpus of data and then fine-tuned on a smaller dataset specific to the task at hand.
Once the model has been fine-tuned, it can be translated from one language to another. The input to the model is a source sentence in one language, and the output is a translation of that sentence in another. The model can be used for both machine and human translations.
One of the advantages of using ChatGPT for language translation is its ability to handle a wide range of languages. The pre-trained model has been trained on diverse languages, so it can be fine-tuned to translate between different languages with relatively little data. Additionally, ChatGPT can handle a wide range of grammar and vocabulary, making it well-suited for tasks that involve more complex or idiomatic language.
The fine-tuning process requires a relatively large amount of parallel data to train the model effectively. The amount of data required will vary depending on the specific task and the languages involved, but as a general rule, the more data available, the better the model will perform. It's also worth noting that the data quality is just as important as the quantity. If the data is noisy, contains errors, or is well-aligned, it will negatively impact the performance of the fine-tuned model.
One can use various metrics to evaluate a ChatGPT language translation model's performance, such as the BLEU score, METEOR, or ROUGE score. BLEU score is a commonly used metric that compares the output of the model to reference translations. The higher the BLEU score, the more similar the output is to the reference translations. METEOR is another commonly used metric that measures the overall quality of the translation, taking into account fluency, grammaticality, and meaning preservation. ROUGE is a metric that evaluates the overlap of the model's output with the reference translations.
In addition, it's important to note that the model may not always produce the best result; it's important to evaluate the model's performance using metrics such as BLEU score, METEOR, or ROUGE score. One should also consider the real-world use case, such as the translation's context, audience, and purpose. The model can be fine-tuned with specific data for certain industries, such as legal or medical, to improve its performance in those fields.
The following are six ways to use ChatGPT for language translation:
The fine-tuning process begins by selecting a parallel corpus of text data in the source and target languages. A parallel corpus is a collection of sentences or documents in the source language and their corresponding translations in the target language. The quality and quantity of the parallel corpus will greatly impact the performance of the fine-tuned model. It's important to ensure that the data is well-aligned, high-quality, and relevant to the task. Once the parallel corpus is selected, the pre-trained model is fine-tuned on this data by adjusting the model's parameters to minimize the difference between the model's output and the target translations. The fine-tuning process uses a backpropagation algorithm to update the model's parameters based on the differences between the model's output and the target translations.
The fine-tuned model can then translate new text from one language to another. The input to the model is a sentence or a document in the source language, and the output is the translation of that sentence or document in the target language.
One of the advantages of using ChatGPT for language translation is its ability to handle a wide range of languages and text types. The pre-trained model has been trained on diverse texts, so it can be fine-tuned to translate a wide range of languages, such as English, Spanish, Chinese, etc. Additionally, ChatGPT can understand the context and meaning of the text, making it well-suited for tasks that involve more complex or idiomatic language.
The fine-tuned model uses the attention mechanism to translate the text. The attention mechanism is a technique used in neural networks to focus on the most relevant parts of the input when making predictions. In the case of language translation, the attention mechanism allows the model to focus on the most relevant parts of the source text when generating the target translation.
Another advantage of using ChatGPT for language translation is that it's a fully neural network based, which allows it to generalize to unseen text, as it can learn the underlying patterns and relationships between words, phrases, and sentences. This makes the model capable of understanding the context and meaning of the text, which is important in translation. It's important to note that the model may not always produce the best result, and it's important to evaluate the model's performance using metrics such as BLEU score, METEOR, or TER. One should also consider the real-world use case, such as the translation's context, audience, and purpose. The model can be fine-tuned with specific data for certain industries, such as legal or medical, to improve its performance in those fields.
In conclusion, ChatGPT can translate text from one language to another by fine-tuning the pre-trained model on a dataset of parallel text in different languages. The fine-tuning process requires a relatively large amount of parallel data, and the quality of the fine-tuned model will depend on the quality and quantity of the data used for fine-tuning.
Generate text that appears to have been written by a human: ChatGPT can be used to generate text that appears to have been written by a human. Applications such as chatbots, content production, and games based on language could all benefit from this feature. Now how does this work?
ChatGPT is a large language model that has been pre-trained on a massive amount of text data, which allows it to generate text that appears to have been written by a human. This is achieved through the use of a technique called unsupervised learning, where the model learns patterns and relationships in the text data without explicit instruction.
The pre-training begins by feeding the model a large corpus of text data, such as books, articles, and websites. The model is then trained to predict the next word in a sentence, given the previous words. During this process, the model learns to understand the context and meaning of the text, and it begins to form its understanding of the relationships between words and phrases. After pre-training, the model can be fine-tuned on a smaller dataset specific to a particular task, such as language translation or text summarization. Fine-tuning is adjusting the model's parameters to minimize the difference between the model's output and the target output. This allows the model to learn the specific patterns and relationships relevant to the task.
Once fine-tuned, the model can generate text that appears to have been written by a human. The model takes a prompt, or starting text, as input and generates text that continues the story, conversation, or information provided in the prompt. The generated text is coherent and grammatically correct and incorporates the prompt's context and meaning.
One of the advantages of using ChatGPT for text generation is its ability to handle a wide range of text types and styles. The pre-trained model has been trained on diverse texts, so it can be fine-tuned to generate text in various styles, such as news articles, poetry, or dialogue. Additionally, ChatGPT can understand the context and meaning of the text, making it well-suited for tasks that involve more complex or idiomatic language.
ChatGPT for text generation is fully based on a neural network, which allows it to generalize to unseen text. The model can learn the underlying patterns and relationships between words, phrases, and sentences, which allows it to understand the context and meaning of the text. This makes the model capable of understanding the context and meaning of the text, which is important in text generation.
In conclusion, ChatGPT generates text that appears to have been written by humans using unsupervised learning. The model is pre-trained on a large corpus of text data and then fine-tuned on a smaller dataset specific to a particular task. The fine-tuning process allows the model to learn the specific patterns and relationships relevant to the task. Once fine-tuned, the model can take a prompt as input and generate text that continues the story, conversation, or information provided in the prompt. The generated text is coherent and grammatically correct and incorporates the prompt's context and meaning. Additionally, ChatGPT can understand the context and meaning of the text, which is important in text generation, and also it can handle a wide range of text types.
Answer Questions: It is possible to utilize ChatGPT to answer questions by examining the query's surrounding context and then offering a response pertinent to the inquiry. When the model is used for question answering, it takes the input question and generates a response. It does this by first encoding the input question into a fixed-length vector representation, called contextual embedding, which captures the meaning of the input. The model then uses this embedding as a starting point to generate the response. ChatGPT can generate human-like responses, as it has been trained on a vast amount of text data. This allows it to understand the question's context and generate a coherent response that makes sense.
Additionally, ChatGPT can also generate responses that are not present in the training data, as it is capable of understanding the context and generating new responses based on what it has learned. ChatGPT can handle a wide variety of question types, such as fact-based, open-ended, and multi-turn questions. This is because the model has learned patterns and relationships in the text data that allow it to understand the context and meaning of the question.
In addition, ChatGPT can handle different languages and answer questions in multiple languages. To do this, the model needs to be fine-tuned on a dataset of questions and answers in the target language. However, it is important to note that the model's ability to answer questions in different languages may vary depending on the quality and quantity of the training data.
ChatGPT is a powerful and versatile language model that can provide answers to a wide variety of questions. Its ability to understand the context and meaning of the question and generate human-like responses makes it a valuable tool for natural language processing tasks. By fine-tuning the model on specific datasets, it can be adapted to handle different question types and languages, allowing it to provide accurate and coherent answers.
Understand the tone of a piece of text: Did you know that you can use ChatGPT to learn to comprehend the tone of a piece of writing, such as whether it is positive, negative, or neutral? Although ChatGPT can identify patterns in text that may indicate the text's tone, it cannot understand the tone of a piece of text in the same way that a human would.
ChatGPT, like most language models, relies on the patterns and relationships that it has learned during its pre-training phase to understand the tone of a piece of text. These patterns are based on the context of the words and phrases used, as well as the context of the entire text. When ChatGPT is presented with a new text, it analyzes the patterns and relationships it learned during its pre-training phase. The model can recognize words and phrases commonly associated with a certain tone. For example, the model may have learned that certain words or phrases, such as "sadly," "regrettably," or "unfortunately," are often associated with a negative tone, while other words or phrases, such as "happily," "delightfully," or "excitingly," are often associated with a positive tone.
Similarly, it can learn that certain sentence structures, punctuation, and capitalization are commonly used in a formal or informal tone. It can also use the context of the entire text to understand the tone. For example, if a text discusses a serious topic, such as a natural disaster or a political crisis, the model may understand that the text has a serious tone. However, if the text discusses a light-hearted topic, such as a comedy show or a vacation, the model may understand that the text has a more casual and light-hearted tone.
It is also important to note that the model's understanding of the tone of a text will depend on the quality and quantity of the training data. If the model has been trained on a diverse set of text with different tones, it will likely have a better understanding of tone than if it has only been trained on a limited text set. However, it is important to note that the model's understanding of the tone of a text will depend on the quality and quantity of the training data. If the model has been trained on a diverse set of text with different tones, it will likely have a better understanding of tone than if it has only been trained on a limited text set. Additionally, ChatGPT, a machine learning model, may need help understanding human emotions' nuances, subtleties, and complexities.
In summary, ChatGPT uses the patterns and relationships that it has learned during its pre-training phase to understand the tone of a piece of text. It can recognize the tone of a text by analyzing the context of the words and phrases used and the context of the entire text. However, its ability to understand the tone of a text may vary depending on the quality and quantity of the training data. Finally, while ChatGPT can be used to identify patterns in text that may indicate the text's tone, it cannot understand the tone in the same way that a human would and can only be used as an approximation.
Generate creative texts: You can use ChatGPT to generate creative texts such as poetry, songs, short stories, and even headlines for newspapers and magazines. ChatGPT can generate text that appears to have been written by a human, and it can be used to generate creative texts, such as poetry, short stories, and even entire novels. ChatGPT, like other language models, uses deep learning to generate text. The model is trained on a large dataset of text and learns patterns and relationships within the text. This allows the model to generate text similar to the training data.
The model uses the patterns and relationships it learned during pre-training to generate text similar to the training data. When given a prompt or a starting point, it can generate text that continues the story or resembles the style and tone of the provided prompt. When generating creative texts, such as poetry, short stories, and novels, ChatGPT uses a process called "text generation." The process starts with a prompt, a short text that provides a starting point for the generation process. The prompt can be a sentence, a phrase, or even a single word. The model then uses the patterns and relationships it has learned from the training data to generate text that continues the story or resembles the style and tone of the provided prompt. The text generation process can be further fine-tuned by adjusting the model's parameters, such as the temperature, which controls the randomness of the generated text and length.
It's important to note that the quality and diversity of the training data play an important role in the generation of creative text. If the model has been trained on a diverse set of creative texts, it will likely generate more creative and diverse text than if it has been trained on a limited set of text. Additionally, the ability of ChatGPT to generate creative text will depend on the specific task and use case. It is important to note that ChatGPT is a machine learning model, and its ability to generate creative texts will depend on the quality and quantity of the training data. If the model has been trained on a diverse set of creative texts, it will likely generate more creative and diverse text than if it has been trained on a limited text set. Additionally, it's important to note that the text generated by ChatGPT may be somewhat original or creative since it is based on patterns and relationships learned from the training data.
In summary, ChatGPT generates creative texts using a technique called "text generation," where it starts with a prompt and uses the patterns and relationships it has learned from the training data to generate text that continues the story or resembles the style and tone of the provided prompt. The quality and diversity of the training data, the specific use case, and the specific task will determine the quality of the generated creative text.
Generation of dialogue: ChatGPT, which stands for "Conversational Generative Pre-training Transformer," uses a type of machine learning called deep learning to generate dialogue. Specifically, it uses a variant of the transformer architecture, which is a type of neural network well-suited for handling sequential data such as text. "Generation of dialogue" refers to the ability of ChatGPT to create a conversation between characters in various contexts. This can include dialogues in video games, movies, or chatbots. ChatGPT uses machine learning techniques to generate realistic and coherent conversations, which can be customized for different situations and settings. This can be done in different contexts, such as genres, languages, or scenarios. Overall, the goal is to create realistic and engaging dialogues that can be used in various applications, such as entertainment or customer service. ChatGPT can generate dialogues between characters in games, movies, or chatbots.
When generating dialogue, ChatGPT is trained on a large dataset of existing text, such as movie scripts, books, and conversation logs. It learns patterns and structures in the language, which allows it to generate new, coherent, and contextually appropriate text.
The basic process of generating dialogue with ChatGPT involves providing the model with a prompt, a piece of text that sets the context or topic for the conversation. The model generates a response, a continuation of the prompt, or a new statement. The model uses the context provided by the prompt and its internal knowledge to generate a coherent and contextually appropriate response.
It can also be fine-tuned with specific task-related data to achieve more specific and accurate dialogue generation. This fine-tuning allows the model to adapt to different scenarios and settings, such as customer service or role-playing games. Overall, ChatGPT uses its pre-trained knowledge to generate coherent, contextually appropriate dialogue, and in some cases, even engaging and entertaining.
Summarization
ChatGPT uses natural language processing (NLP) to summarize long articles or documents into shorter forms. This process is also known as "text summarization." By using its pre-trained knowledge of language patterns, ChatGPT can identify and extract the most important information from a document and present it in a condensed form. This can make it easier for people to read and understand the original text's main ideas or key points. The summary generated by ChatGPT is coherent and contextually appropriate; it can be used for different purposes, such as summarizing news articles or condensing legal or technical documents. This way, people can quickly get an overview of the information presented in the original text without reading through the entire document. ChatGPT is a powerful language model that can be fine-tuned for various natural language processing tasks, including summarization. In this section, we will explain how to use ChatGPT for summarization in more detail.
The first step in using ChatGPT for summarization is to fine-tune the pre-trained model on a dataset of documents and their corresponding summaries. This fine-tuning process can be done using a technique called transfer learning, where the model is pre-trained on a large corpus of data and then fine-tuned on a smaller dataset specific to the task at hand. Once the model has been fine-tuned, it can generate summaries of new documents. The input to the model is a document, and the output is a summary of the document. The model can be used for both machine and human summaries.
One of the advantages of using ChatGPT for summarization is its ability to handle a wide range of text types and formats. The pre-trained model has been trained on diverse texts, so it can be fine-tuned to summarize a wide range of documents, such as news articles, scientific papers, and legal documents. Additionally, ChatGPT can understand the context and meaning of the text, making it well-suited for tasks that involve more complex or idiomatic language.
The fine-tuning process requires a relatively large amount of data to train the model effectively. The amount of data required will vary depending on the specific task and the type of texts involved, but as a general rule, the more data available, the better the model will perform. It's also worth noting that the quality of the data is just as important as the quantity. If the data is noisy, contains errors, or is well-aligned, it will positively impact the performance of the fine-tuned model.
To evaluate the performance of a ChatGPT summarization model's performance, one can use various metrics, such as ROUGE score, METEOR, or CIDEr. ROUGE score is a commonly used metric that compares the output of the model to reference summaries by measuring the overlap of the model's output with the reference summaries. METEOR is another commonly used metric that measures the overall quality of the summary, taking into account fluency, grammaticality, and meaning preservation. CIDEr is a metric that measures the similarity between the reference and generated summaries.
In addition, it's important to note that the model may only sometimes produce the best result. It's important to evaluate the model's performance using metrics such as ROUGE score, METEOR, or CIDEr. One should also consider the real-world use case, such as the summarization's context, audience, and purpose. The model can be fine-tuned with specific data for certain industries, such as legal or medical, to improve its performance in those fields.
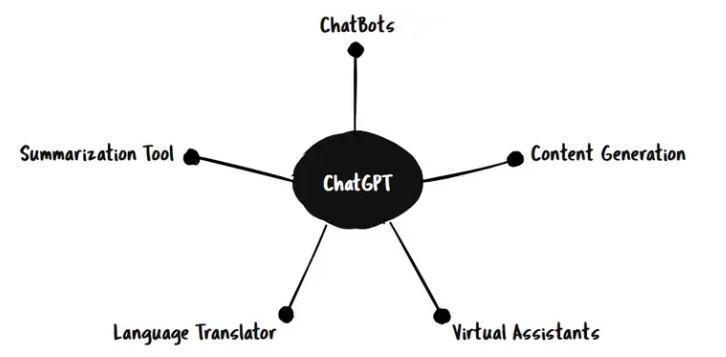
Use cases of ChatGPT.
Source: Biswas, D. CHATGPT, and its implications for enterprise AI, LinkedIn.
In conclusion, ChatGPT is an effective language model that can be customized to perform a wide range of natural language processing tasks, such as the translation and summarization of linguistic data. However, fine-tuning takes a substantial amount of training data, and the quality of the fine-tuned model will rely on the quality and quantity of the data used for fine-tuning. Fine-tuning is a relatively time-consuming process. In addition, the model could not always deliver the optimal outcome; therefore, it is essential to evaluate the model's performance using metrics relevant to the situation.
ChatGPT is a powerful language model that can be fine-tuned for language translation tasks. The fine-tuning process requires a relatively large amount of parallel data, and the quality of the fine-tuned model will depend on the quality and quantity of the data used for fine-tuning. Additionally, it's important to evaluate the model's performance using appropriate metrics, such as the BLEU score. It's important to note that fine-tuning a pre-trained model like ChatGPT on a specific task requires a relatively large amount of training data, and the quality of the fine-tuned model will depend on the quality and quantity of the data used for fine-tuning. Additionally, the model may not always produce the best result, and it's important to evaluate the model's performance using metrics such as BLEU score, METEOR, or ROUGE score.
Resources:
Jiao, W., Wang, W., Huang, J., Wang, X., & Tu, Z. (2023). Is ChatGPT a Good Translator? ArXiv; A preliminary Study.
I translated my article on ChatGPT using ChatGPT, what do you think of the result? Marengo
The “Transformative Innovation” series is for your reading-listening pleasure. Order your copies today!
Regards, Genesys Digital (Amazon Author Page) https://tinyurl.com/hh7bf4m9